- 1Microbiome Study Group, School of Health Sciences Dr. Paulo Prata (FACISB), Barretos, Brazil
- 2QGene-Solutions and Logistics in Health, Sao Carlos, Brazil
- 3Board of Health from Barretos, Barretos, Brazil
- 4Department of Technology, School of Agricultural and Veterinarian Sciences, São Paulo State University (UNESP), Jaboticabal, Sao Paulo, Brazil
- 5DNA Consult Genetics and Biotechnology, Sao Carlos, Brazil
- 6Biotechnology Department, Sao Carlos Federal University, UFSCAR, Sao Carlos, Brazil
- 7Barretos Cancer Hospital (HCB), Barretos, Brazil
- 8Institute of Biosciences, Humanities and Exact Sciences (IBILCE), São Paulo State University (UNESP), Sao Jose do Rio Preto, Sao Paulo, Brazil
Intestinal dysbiosis associated with immunological deregulation, leaky gut, bacterial translocation, and systemic inflammation has been associated with autoimmune diseases, such as type 1 diabetes (T1D). The aim of this study was to investigate the intestinal dysbiosis in T1D patients and correlate these results with clinical parameters and cytokines. The present study was approved by the Barretos Cancer Hospital (Process number 903/2014), and all participants have signed the informed consent in accordance with the Declaration of Helsinki, and answered a questionnaire about dietary habits. Stool samples were used for bacterial 16S sequencing by MiSeq Illumina platform. IL-2, IL-4, IL-6, IL-10, IL-17A, TNF, and IFN-γ plasma concentrations were determined by cytometric bead arrays. The Pearson’s chi-square, Mann–Whitney and Spearman correlation were used for statistical analyses. Alpha and beta diversities were conducted by using an annotated observed taxonomic units table. This study included 20 patients and 28 controls, and we found significant differences (P < 0.05) among consumption of vegetables, proteins, milk and derivatives, spicy food, and canned food when we compare patients and controls. We detected intestinal dysbiosis in T1D patients when we performed the beta diversity analysis (P = 0.01). The prevalent species found in patients’ stool were the Gram-negatives Bacteroides vulgatus, Bacteroides rodentium, Prevotella copri, and Bacteroides xylanisolvens. The inflammatory interleukin-6 was significantly increased (P = 0.017) in patients’ plasma. Furthermore, we showed correlation among patients with poor glycemic control, represented by high levels of HbA1C percentages and Bacteroidetes, Lactobacillales, and Bacteroides dorei relative abundances. We concluded that there are different gut microbiota profiles between T1D patients and healthy controls. The prevalent Gram-negative species in T1D patients could be involved in the leaky gut, bacterial translocation, and poor glycemic control. However, additional studies, with larger cohorts, are required to determine a “signature” of the intestinal microbiota in T1D patients in the Brazilian population.
Introduction
Type 1 diabetes (T1D) or autoimmune diabetes is a chronic disease mediated by immune reactions against pancreatic beta cells, resulting in insulin dependence to regulate blood glucose concentrations (1). The T1D pathogenesis involves the interaction of genetic and environmental factors, such as viral infections, vitamin deficiencies, and intestinal dysbiosis (2). According to the International Diabetes Federation, more than 96,000 children and adolescents under 15 years will be diagnosed with autoimmune diabetes annually worldwide (3).
The gut microbiota might modulate the T1D pathogenesis via two mechanisms. In the first step, an impaired tolerance process in infancy can predispose to develop autoimmune diseases, such as T1D, and might induce autoreactive T cell activation and autoantibodies (4). At the second step, intestinal dysbiosis may lead children with genetic predisposition and positive autoantibodies to develop clinical disease (4).
Studies in animal models reported an increased relative abundance of Bacteroides, Ruminococcus, and Eubacterium genera in biobreeding diabetes-prone rats, and an increased abundance of Bifidobacterium and Lactobacillus in diabetes-resistant rats (5). Also, the prevalence of the Bacteroidetes phylum members could promote increased intestinal permeability and precede the clinical onset of T1D in animal models of the disease, in pre-diabetic patients and in diabetic subjects (6, 7).
In T1D children, there is decreased gut microbiota diversity and abundance of mucin-degrading and butyrate-producing members, and reduced Firmicutes/Bacteroidetes ratio, along with Lactobacillus, Bifidobacterium, and Prevotela species (8). Smaller relative abundance of lactate-producing bacteria, including the Bifidobacterium longum, and increased Clostridium, Bacteroides, and Veillonella species are equally detected (9).
Moreover, intestinal dysbiosis was detected in pre-diabetic children with genetic predisposition and beta cells autoantibodies (10). Increased Bacteroides dorei and Bacteroides vulgatus species in seroconverted T1D patients are registered 8 months prior to beta cell autoimmunity, suggesting that early dysbiosis may predict T1D in genetically predisposal subjects (11). Additionally, children with beta cell autoantibodies exhibited increased Bacteroidetes members and decreased lactate and butyrate-producing bacteria (12). These studies support the hypothesis that there is a gut microbiome signature associated with T1D development in seropositive children (13).
Previous reports suggested the involvement of the gut mucosa in the pathogenesis of islet autoimmunity in T1D (14). Thus, the modulation of interactions between commensal microbiota and gut-associated lymphoid tissues may represent a means to affect the progression of the autoimmune diabetes (15). Based on previous studies that identify that dysbiosis may be strongly correlated with barrier disruption, bacterial translocation, and autoimmune diseases development (16), we hypothesized that the prevalence of Gram-negative bacteria in the gut mucosa from T1D patients is greater in patients than in stool samples from controls, and positively correlated with poor glycemic control and systemic inflammatory cytokines. In the present study, we investigate the intestinal dysbiosis in T1D patients and correlated these results with clinical data and systemic inflammatory cytokines.
Materials and Methods
Patients and Controls Enrollment
Type 1 diabetes patients with fasting blood glucose greater than 126 mg/dL (3) at diagnosis were enrolled by the physician from the endocrinology department from Board of Health from Barretos, Sao Paulo, Brazil, from June 1st, 2015 to July 30th, 2016. A total of 20 patients, 14 females and 6 males (mean age ± SD = 23.1 ± 8.6 years), were included in the present study.
Twenty-eight healthy subjects, 18 females and 10 males (mean age ± SD = 25.2 ± 9.8 years), were enrolled in the present study that was performed in accordance with the recommendations of Ethics committee from Barretos Cancer Hospital. All participants have signed the informed consent in accordance with the Declaration of Helsinki. The present study was approved by the Barretos Cancer Hospital (Process number 903/2014). After the consent, the peripheral blood was collected and stool samples were delivered within 5 days. Stool and plasma samples were stored at −80°C until DNA extraction and cytokine quantification.
At enrollment, all of the subjects answered a questionnaire about dietary habits, such as daily consumption of vegetables, fresh fruits, carbohydrates, proteins (meat/eggs), trans fat, milk and derivatives, spicy food, canned food, hot drinks (coffee/tea), and alcohol. Exclusion criteria include use of anti-inflammatories, antibiotics, laxatives, vaccination, and corticosteroids in the last 30 days. Chronic diarrheas and gastrointestinal surgeries were also considered as exclusion criteria for patients and controls.
Anthropometric measurements and clinical data from T1D patients, such as weight, height, fasting blood glucose and glycated hemoglobin (HbA1c), and disease duration were recorded. The BMI mean was 23.9 ± 3.6 (nine patients presented BMI < 25 and seven patients were overweighed, with BMI > 25). The fasting blood glucose mean was 236.8 ± 135.9 mg/dL (five patients with controlled blood glucose < 126 mg/dL, 11 patients with uncontrolled blood glucose > 126 mg/dL, and four patients without this clinical data). The HbA1C mean was 9.8 ± 1.8% (13 patients with poor glycemic control, HbA1C > 7.5%, one patient with moderate glycemic control, HbA1C = 7%, and six patients without this clinical data). The disease duration mean was 14.0 ± 7.2 years. Demographic characteristics, anthropometric measurements, and clinical data from T1D patients, were summarized in Table 1.
DNA Extraction and Bacterial 16S Sequencing
DNA was extracted from 250 mg from stool samples by using PowerSoil DNA Isolation Kit (MO BIO Laboratories, QIAGEN, CA, USA), according to manufacturer’s instructions. DNA quantification was performed by Quantus fluorometer and adjusted to 5 ng/mL with Tris buffer (10 mM, pH 8.5). V3 and V4 regions of the bacterial 16S (17) (Forward primer: 5′-TCGTCGGCAGCGTCAGATGTGTATAAGAGACAGCCTACGGGNGGCWGCAG; Reverse primer: 5′GTCTCGTGGGCTCGGAGATGTGTATAAGAGACAGGACTACHVGGGTATCTAATCC) were amplified by PCR, using bacterial DNA (2.5 mL), primers (5 mL), and 12.5 mL of 2× KAPA HiFi HotStart Ready Mix (Kapa Biosystems, MA, USA). Purification of PCR products was performed by AMPure XP Beads Kit (BD Biosciences, CA, USA). DNA libraries were constructed according to the Illumina protocols, according to the workflow represented in Figure 1. The sequencing was performed by using an Illumina MiSeq platform system (MiSeq Desktop Sequencer).
Cytokine Determination by Cytometric Bead Array
Peripheral blood (10 mL) was collected from T1D patients and controls and plasma-EDTA was isolated by centrifugation at 1,372 g, for 10 min, 4°C. Cytokine detection was performed by cytometric bead array (Human Th1/Th2/Th17 Cytokine Kit, BD Biosciences, CA, USA). Plasma levels of IL-2, IL-4, IL-6, IL-10, IL-17A, TNF, and IFN-γ were determined by flow cytometer FACSCanto™ II (BD Biosciences). Analyses was performed by BDFCAP array™ software and data were expressed by conversion of the median fluorescence intensity in picogram per milliliter.
Statistical Analyses
Data extracted from the questionnaires containing dietary habits were analyzed by Pearson’s chi-square. Comparisons between cytokines plasma concentrations in T1D patients and controls subjects were performed by Mann–Whitney. Correlations among reads percentages of the intestinal microbiota, cytokines plasma concentrations, and clinical data were performed by Spearman correlation. Analyses of variance, diversity indexes, and alpha and beta diversities were performed by using annotated Operational Taxonomic Units table. Sequencing analyses of the bacterial 16S was performed as described in our previous work (18). P values less than 0.05 were considered statistically significant.
Results
Dietary Habits and Correlations With the Gut Microbiota in T1D Patients
To evaluate the dietary habits in T1D patients and healthy controls, we applied a questionnaire about daily consumption of vegetables, fresh fruit, carbohydrates, proteins, trans fat, milk and derivatives, spicy food, canned food, hot drinks (coffee/tea), and alcohol. Regarding these variables, subjects reported daily consumption of vegetables [patients (P) = 37.5%; controls (C) = 63%], fresh fruits [P = 29.2%; C = 29.6%, carbohydrates (P = 45.8%; C = 44.4%), proteins (meat/eggs) (P = 41.7%; C = 48.1%), trans fat (P = 25%; C = 29.6%), milk and derivatives (P = 45.8%; C = 55.6%), spicy food (P = 8.3%; C = 11.1%), canned food (P = 4.2%; C = 0%), hot drinks (P = 66.7%; C = 81.5%), and alcohol (P = 50%; C = 59.3%)]. We observed significant differences (P < 0.05) among consumption of vegetables, proteins, milk and derivatives, spicy food, and canned food (Figure 2).
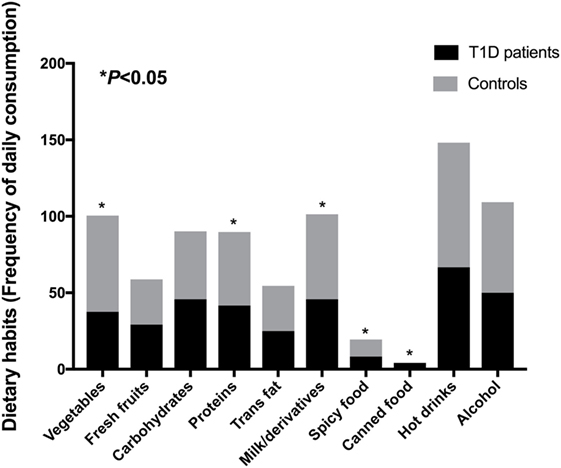
Figure 2. Dietary habits from T1D patients and control subjects. Statistical analyses were performed by Pearson’s chi-square. Significance was set at P < 0.05. Horizontal bars represent percentages of positive answer for variables derived from the questionnaire.
To find correlations between dietary habits and microbial community, we analyzed the correlations among consumption of vegetables, fresh fruit, carbohydrates, proteins, trans fat, milk and derivatives, spicy food, canned food, hot drinks, and alcohol with relative abundances of the gut microbiota detected in stool samples from T1D patients. There were correlations between vegetables intake by patients and Clostridia (P = 0.012; r = −0.62) and Clostridiales relative abundances (P = 0.002; r = −0.74), canned food consumption and Bacteroidetes (P = 0.002; r = 0.73), and Flavobacterium (P < 0.001; r = 0.81) relative abundances. Furthermore, there is an inverse correlation between fresh fruits intake and Bacteroides (P = 0.016; r = −0.59), B. vulgatus (P = 0.006; r = −0.67), and Bacteroides rodentium (P < 0.001; r = −0.81) relative abundances, and between protein intake and Clostridiaceae (P = 0.036; r = −0.52), Oscillospira, and Oscillospira eae (P = 0.006; r = −0.68) relative abundances. We also found correlation between hot drinks intake by patients and B. rodentium (P = 0.017; r = 0.59) abundance, and between milk and derivatives consumption with Akkermansia muciniphila (P = 0.016; r = −0.59) relative abundance.
Detection of Intestinal Dysbiosis in T1D Patients
To investigate the dysbiosis in T1D patients, we sequenced the 16S bacterial DNA stool samples from patients and controls and calculated alpha and beta diversities. According to the rarefaction curves, there were no significant differences (P = 0.318) in richness and evenness between samples obtained for patients and controls (Figures 3A,B; Table 2). However, we detected significant differences (P = 0.01) between microbial community found in T1D patients and controls, when we performed the weighted and unweighted UniFrac metric with Bonferroni’s correction analyses (Figures 3C,D).
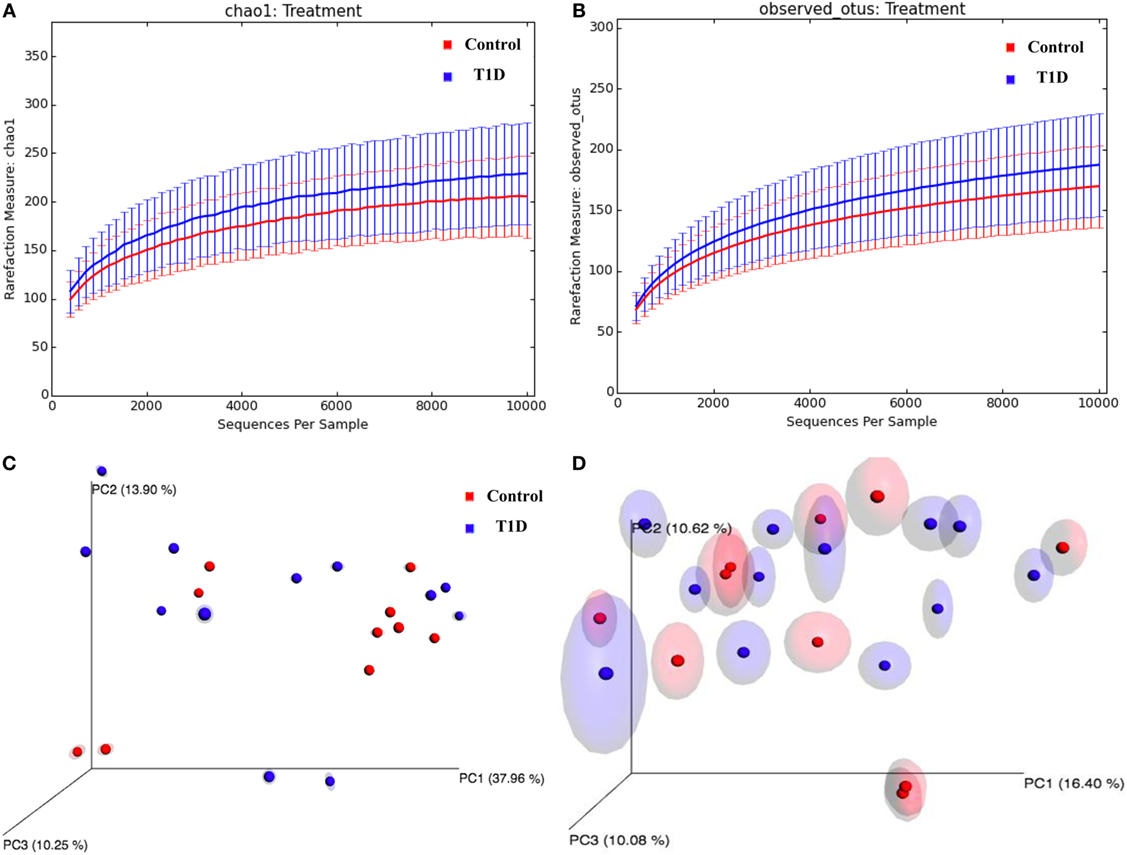
Figure 3. Alpha and beta-diversity in gut microbiota from T1D patients. (A,B) Rarefaction curves comparing the species richness, Chao1, and OTU numbers. (C,D) PcoA plots with weighted and unweighted UniFrac metric with Bonferroni’s correction.
Prevalence of Gram-Negatives in the Gut Microbiota in T1D Patients
To evaluate microbiota composition in stool samples from patients and controls, we compare the reads percentages in both groups. The prevalent phyla in T1D patients were Firmicutes [patient reads (PR) = 47.62%; control reads (CR) = 43.23%] and Bacteroidetes (PR = 43.13%; CR = 45.44%), the prevalent classes were Bacteroidia (PR = 42.73%; CR = 44.52%) and Clostridia (PR = 39.50%; CR = 35.01%), the prevalent orders were Bacteroidales (PR = 42.73%; CR = 44.52%) and Clostridiales (PR = 39.50%; CR = 35.00%), and the prevalent families were Bacteroidaceae (PR = 23.78%; CR = 28.91%), Ruminococcaceae (PR = 19.32%; CR = 13.42%), and Lachnospiraceae (PR = 14.83%; CR = 15.04%) (Figures 4A–D). The prevalent genera in T1D patients were Bacteroides (PR = 23.78%; CR = 28.91%), Alistipes (PR = 7.12%; CR = 6.32%), and Prevotella (PR = 4.50%; CR = 1.89%). The prevalent species in the feces of T1D patients were B. vulgatus (PR = 4.96%; CR = 2.69%), Blautia coccoides (PR = 2.06%; CR = 2.85%), B. rodentium (PR = 2.03%; CR = 3.25%), Prevotella copri (PR = 1.72%; CR = 0.25%), Oscillospira eae (PR = 1.71%; CR = 0.41%), A. muciniphila (PR = 1.61%; CR = 1.82%), and Bacteroides xylanisolvens (PR = 1.23%; CR = 1.09%) (Figures 4E,F). Additionally, we detected significant differences in relative abundance of Betaproteobacteria class (P = 0.021), Clostridiales order (P = 0.007), Ruminococcaceae (P = 0.027) and Lachnospiraceae (P = 0.008) families, and in Escherichia/Shiguela (P = 0.005), Bifidobacterium (P = 0.021), Parabacteroides (P = 0.021), Streptococcus (P = 0.033), Bacteroides (P = 0.038), and Clostridium (P = 0.043) genera (Figure 4).
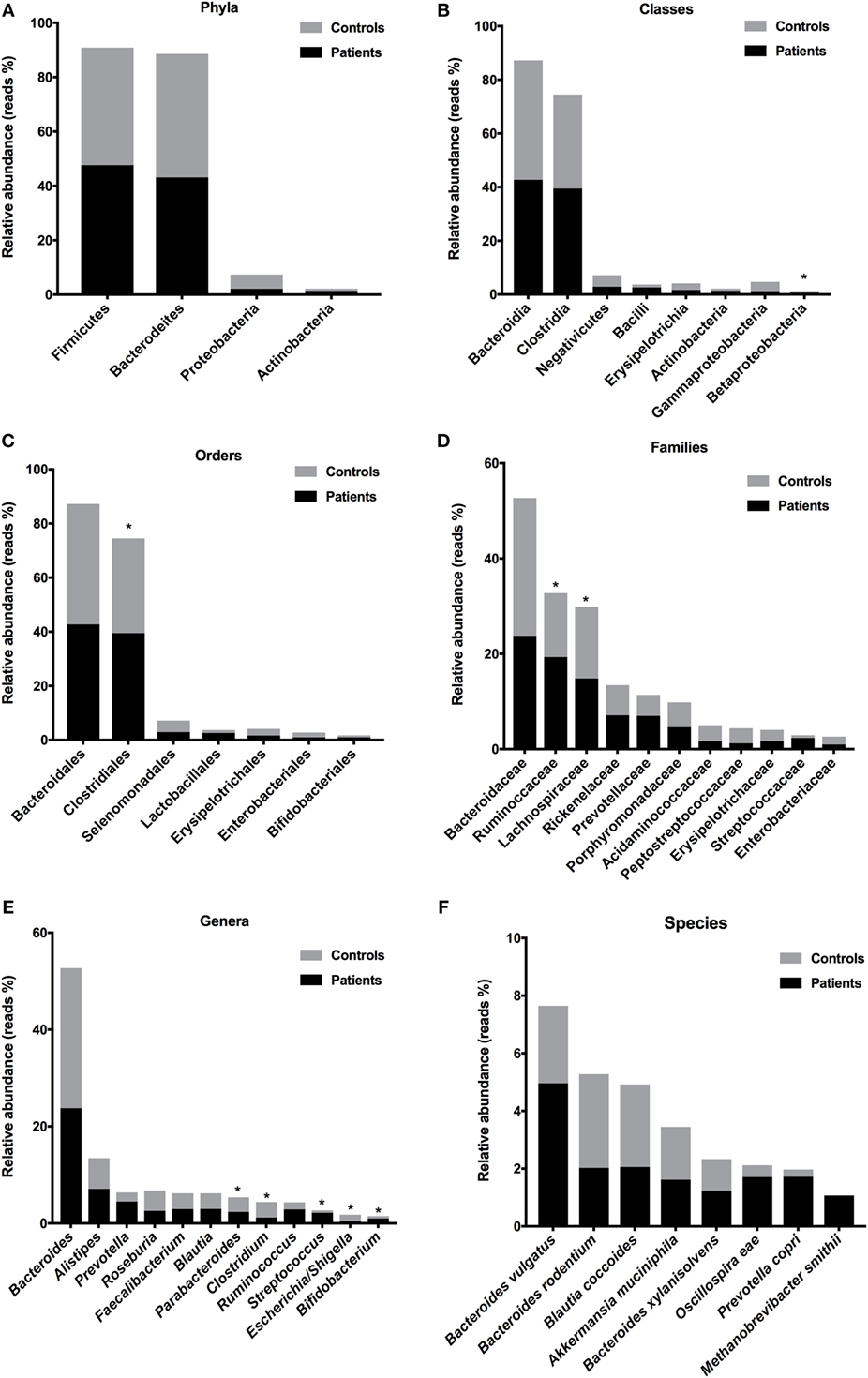
Figure 4. Relative abundances of bacterial taxa in stool samples from T1D patients. Predominant phyla (A), classes (B), orders (C), families (D), genera (E), and species (F). Bars represent the reads percentages found in metagenomics analyses. *P < 0.05.
Intestinal Dysbiosis Correlated With Poor Glycemic Control
To find correlations between microbial community and clinical results, we analyzed the correlations among fasting blood glucose, HbA1c, and disease duration with relative abundances of the gut microbiota detected in stool samples from T1D patients. There was a negative correlation (P = 0.01, r = −0.61) between Bifidobacterium members with fasting blood glucose in T1D patients (Figure 5A). Moreover, correlations among high levels of HbA1C percentages and Bacteroidetes (P = 0.03, r = 0.56), Lactobacillales (P = 0.004, r = −0.74), and Bacteroides dorei relative abundances (P = 0.01, r = −0.60) were detected (Figures 5B–D). Additionally, there was a negative correlation among disease duration and Proteobacteria (P = 0.002, r = −0.78) and Parabacteroides members (P = 0.006, r = −0.71) (Figures 5E,F).
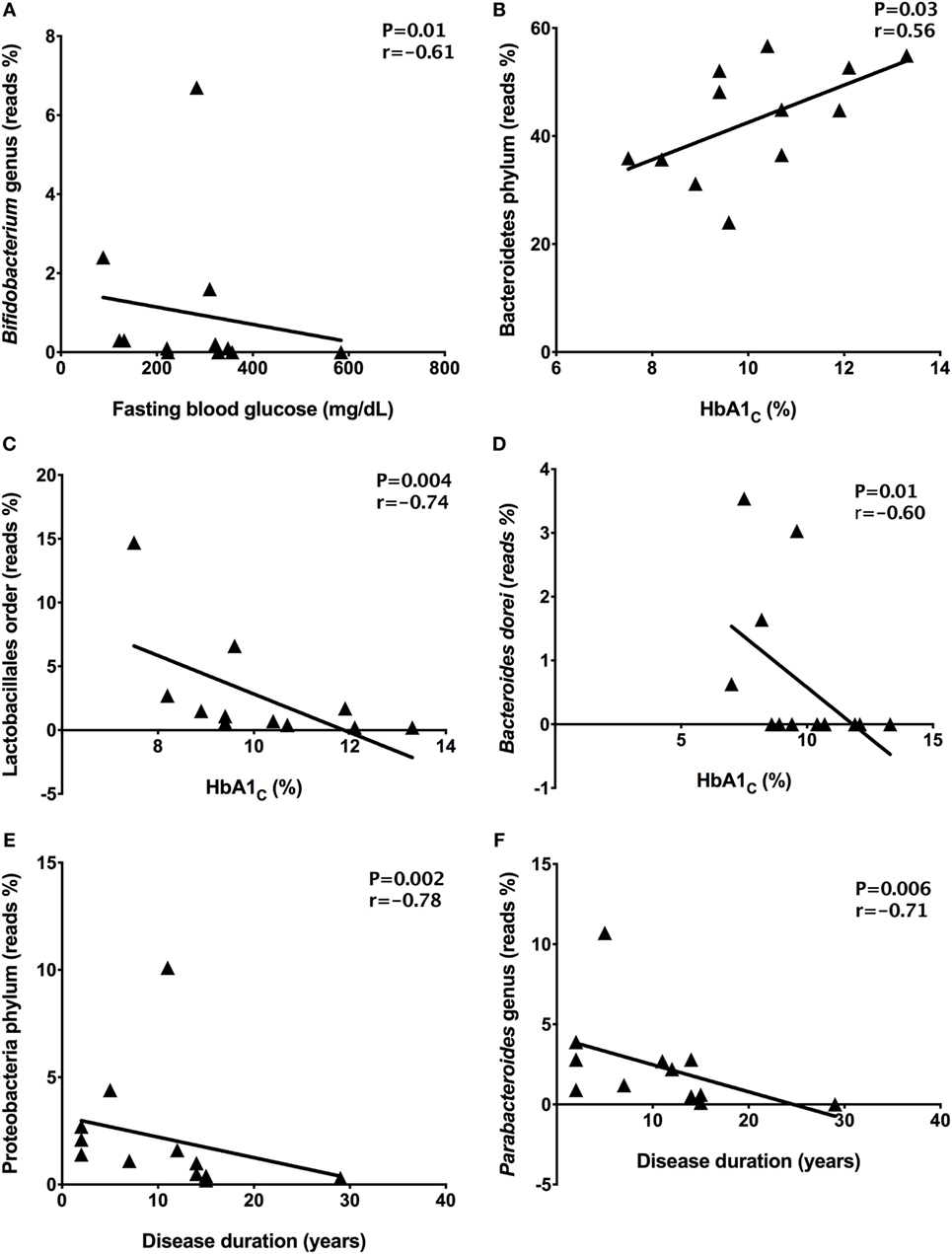
Figure 5. Correlations among relative abundance of bacterial community and clinical data. Bifidobacterium and fasting blood glucose (A), Bacteroidetes and HbA1C (B), Lactobacillales and HbA1C (C), Bacteroides dorei and HbA1C (D), Proteobacteria and disease duration (E), Parabacteroides and disease duration (F). Statistical analyses were performed by using the Spearman’s test. Significance was set at P < 0.05.
Inflammatory Cytokines Positively Correlated With Gram-Negative Bacterial Community
To determine the plasma concentrations of cytokines, we quantified the concentrations of IL-2, IL-4, IL-6, IL-10, IL-17A, IFN-γ, and TNF cytokines by cytometric bead array. IL-2 concentration was undetectable in control samples, and it was impossible to compare with plasma levels obtained for patients (0.308 ± 0.214 pg/mL) (data not shown). The IL-6 plasma concentration was increased (P = 0.017) in patients (1.889 ± 0.268 pg/mL) when compared with control group (1.419 ± 0.387 pg/mL) (Figure 6A). Moreover, there were no significant differences (P > 0.05) in the concentrations of IL-17A, IFN-γ, and TNF inflammatory cytokines in patients’ plasma (IL-17A: 3.445 ± 1.367 pg/mL; IFN-γ: 0.926 ± 0.15 pg/mL; TNF: 0.808 ± 0.304 pg/mL) compared with controls (IL-17A: 2.459 ± 0.713 pg/mL; IFN-γ: 1.046 ± 0.211 pg/mL; TNF: 0.406 ± 0.103 pg/mL) (Figures 6B–D). The IL-4 (P = 0.01) and IL-10 (P = 0.003) plasma concentrations were augmented in patients’ samples (IL-4: 0.434 ± 0.204 pg/mL; IL-10: 0.64 ± 0.206 pg/mL), when compared with healthy subjects (IL-4: 0.014 ± 0.013 pg/mL; IL-10: 0.079 ± 0.032 pg/mL) (Figures 6E,F).
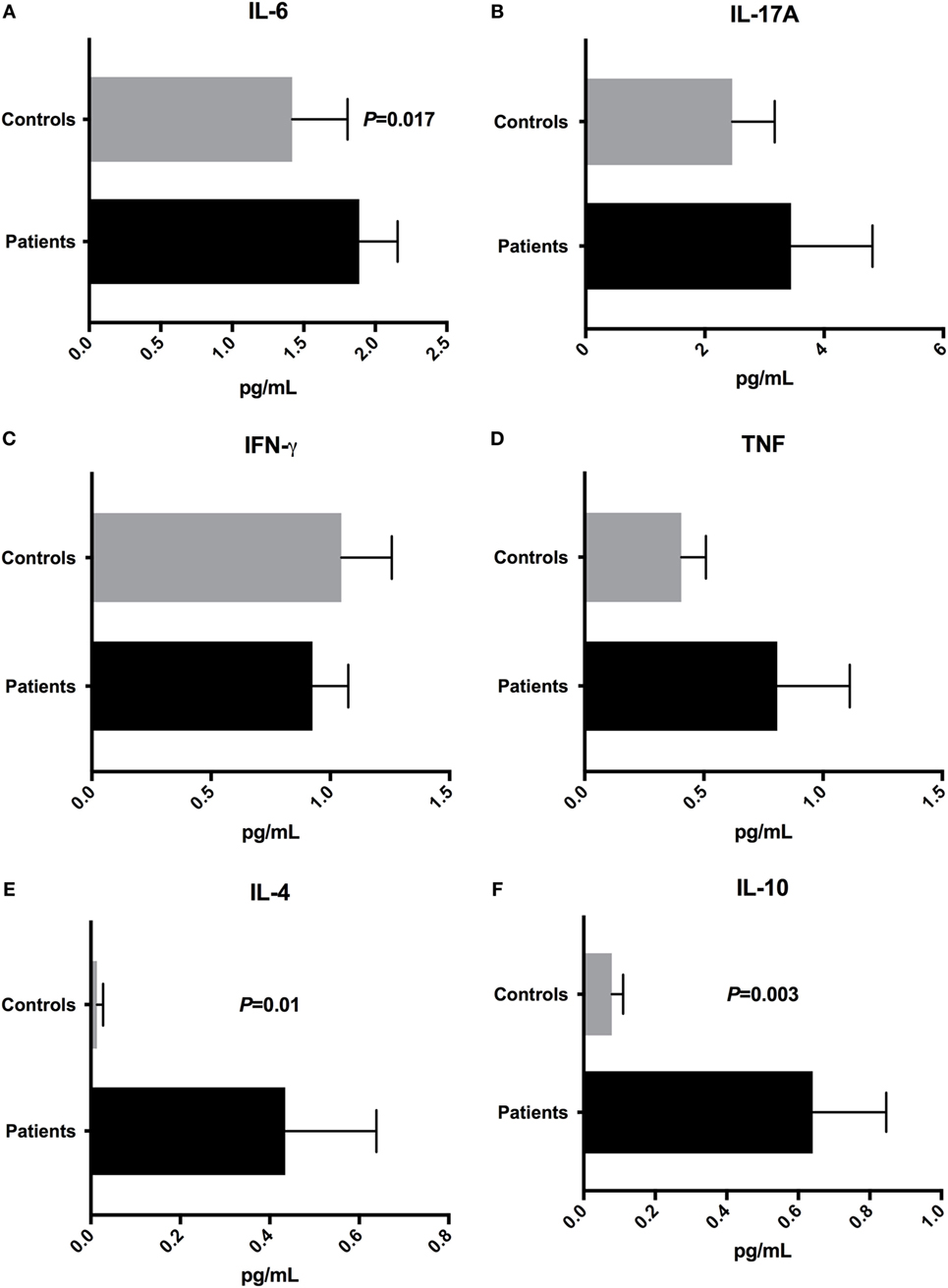
Figure 6. Cytokine profile in T1D patients and control subjects. Plasma concentrations of (A) IL-6, (B) IL-17A, (C) IFN-γ, (D) TNF, (E) IL-4, and (F) IL-10. Statistical analyses were performed by Mann–Whitney test. Significance was set at P < 0.05.
In order to detect correlations between dysbiosis and cytokines, we identified correlations between systemic levels of cytokines and reads percentages of bacterial groups present in stool samples from T1D patients. Significant correlations among the inflammatory cytokine IL-6 and Ruminococcaceae (P = 0.02, r = −0.61) and Ruminococcus members (P = 0.006, r = −0.70) was detected (Figures 7A,B). The TNF plasma levels negatively correlated with Proteobacteria (P = 0.04, r = −0.52) and Clostridiaceae relative abundances (P = 0.02, r = −0.60) (Figures 7C,D). Finally, the IFN-γ (P = 0.008, r = −0.64) and TNF (P = 0.001, r = −0.57) plasma concentrations correlated with relative abundances of B. xylanisolvens (Figures 7E,F).
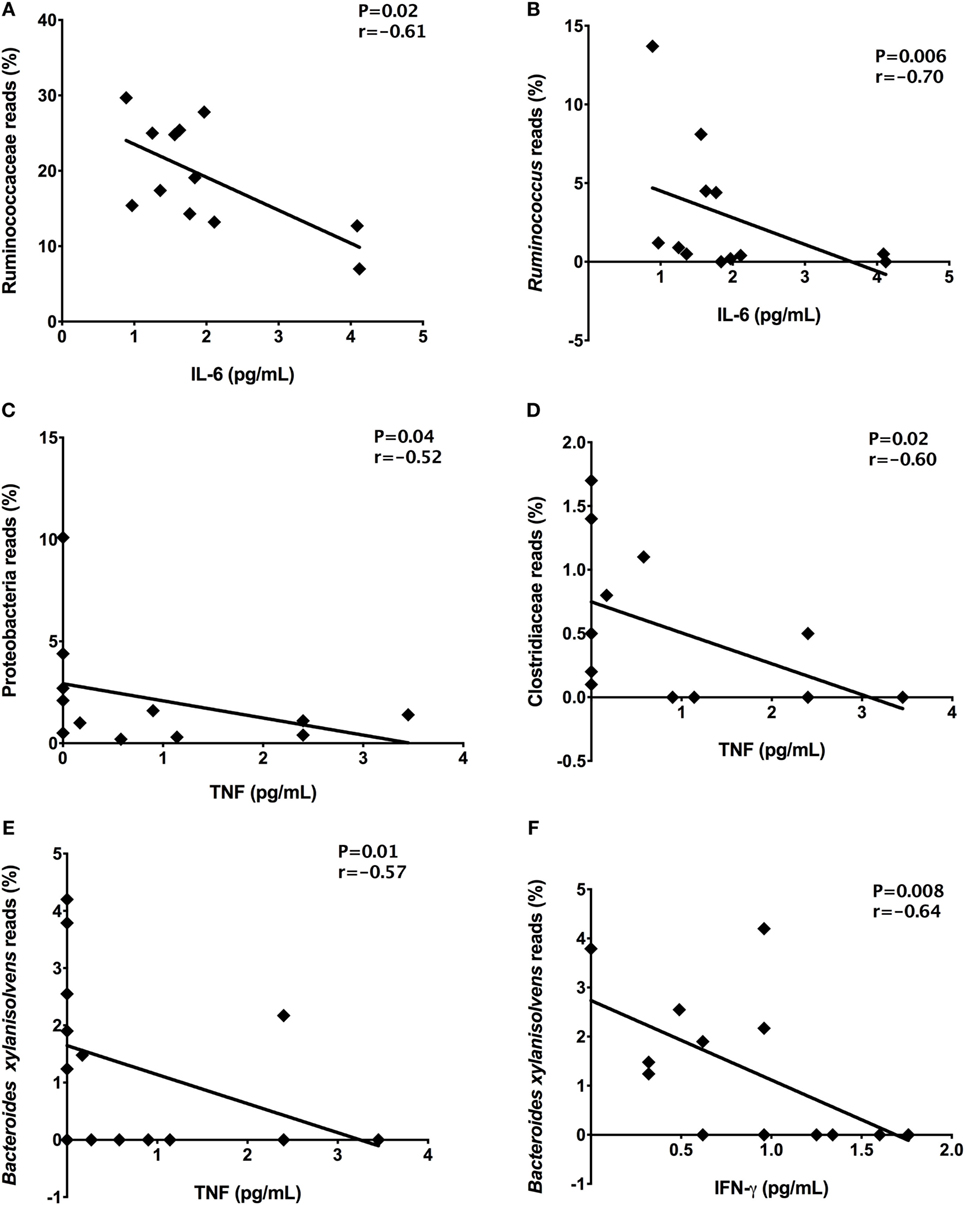
Figure 7. Correlations among the relative abundances of bacterial taxa and plasma concentrations of cytokines. Ruminococcaceae and IL-6 (A), Ruminococcus and IL-6 (B), Proteobacteria and TNF (C), Clostridiaceae and TNF (D), Bacteroides xylanisolvens and TNF (E), Bacteroides xylanisolvens and IFN-γ (F). Statistical analyses were performed by Spearman’s test. Significance was set at P < 0.05.
Discussion
The gastrointestinal tract hosts approximately 100 trillions of bacteria that reside in mucosal surfaces and constantly interact with immune cells (14). This microbial community function as microbiological defense barrier, induce antimicrobial peptides secretion and immunological responses that increase mucosal and systemic immunity (19). Previous studies have focused on the role of commensal microbiota in health maintenance and disease development and environmental factors that influence its dynamics (20, 21). The equilibrium between commensal microbiota and host is characterized by microbiota members that improve metabolism and protect against pathobionts and gut inflammation (22). Several evidences suggest that alterations in function and diversity of the gut microbiota might be linked to the development of autoimmune diseases, including T1D (23). The hypotheses proposed to correlate dysbiosis with autoimmune diseases include bystander T-cell activation, molecular mimicry, amplification of autoimmunity by inflammatory milleu, induced by dysbiotic microbiota, and recently proposed, the posttranslational modification of luminal proteins by enzymes from dysbiotic microbiota (24). This altered posttranslational modification of luminal proteins could produce neo-epitopes that may become immunogenic and trigger systemic autoimmunity and autoimmune diseases (24).
Recent reports have demonstrated the influence of dietary habits in the gut microbiota composition (25–28). In the study from Wu and coworkers (2011), they evaluated the association between diet and gut microbiota composition, in a cohort of 98 healthy subjects. Authors reported that the Bacteroides genera was associated with animal protein and saturated fat consumption, while Prevotella genera was linked to carbohydrates and simple sugar intake (27).
In another study, Yamaguchi and colleagues evaluated dietary habits, metabolic markers, and fecal microbiota in 59 T2D patients. In this study, they reported a correlation between high carbohydrates, fat, and protein consumption with increased counts of Clostridium clusters IV and XI. Bifidobacterium species, Lactobacillales, and Bacteroides species were inversely correlated with carbohydrate, protein consumption, and fasting blood glucose, respectively. Authors concluded that low consumption of proteins and carbohydrates favors a healthy gut microbiota and improve glucose tolerance in T2D patients (28). In our work, we detected an inverse correlation between fresh fruits intake and Bacteroides, B. vulgatus, and B. rodentium abundances, and between protein consumption with Clostridiaceae, Oscillospira, and Oscillospira eae relative abundances.
In the present study, we reported an intestinal dysbiosis in T1D patients, with significant differences in the diversity of the gut microbiota, represented by beta-diversity analysis between patients and controls. Study from Murri and colleagues investigated the gut microbiota in 16 T1D children and 16 control subjects and demonstrated an increased numbers of Clostridium, Bacteroides, and Veillonella genera in patients when compared with control counterparts. Furthermore, the Firmicutes/Bacteroidetes ratio and relative abundances of Lactobacillus, Bifidobacterium, and Prevotela members were decreased in T1D patients (9). Moreover, authors showed correlations among Lactobacillus, Bifidobacterium, and Clostridium members with glucose plasma levels (9).
In this study, we detected the prevalence of gram-negative species in stool samples from our T1D patients, including B. vulgatus, B. rodentium, P. copri, and B. xylanisolvens, supporting our hypothesis and suggesting an increase in the bacterial translocation through the epithelial barrier, triggering systemic inflammation, increased oxidative stress, metabolic deregulation, and beta cell destruction (14). Previous studies in animal models showed that gut microbiota translocation to pancreatic lymph nodes triggers NOD2 activation, Th1 and Th17 differentiation, which contribute to inflammatory infiltrate inside the pancreatic islets and T1D development (29).
The adult healthy intestinal microbiota is composed by Firmicutes, Gram-positive members, and Bacteroidetes, Gram-negative members (30). The prevalent species from Firmicutes phylum are Faecalibacterium prausnitzii and Eubacterium rectale/Roseburia species (31). These bacteria produce short-chain fatty acids, including the butyrate, which suppress NF-κB signaling in the intestinal epithelial cells (31, 32). The Bacteroidetes phylum, the second most prevalent in the human intestine, is dominated by Bacteroides and Prevotella species (33). The Prevotella members are able to activate TLR2 receptors and induce Th17 CD4 T cell differentiation. The prevalence of Bacteroides and Prevotella species is associated with gastrointestinal inflammation, triggered mainly by the inflammatory Th17 cytokines (34). Additionally, Prevotella species induce IL-8 and IL-6 release by epithelial cells, inducing Th17 immune responses and neutrophil recruitment (34). Thus, inflammation of the gastrointestinal mucosa, induced by Prevotella species might promote dissemination of inflammatory mediators, barrier dysfunction, and bacterial translocation, which induce and amplify the systemic inflammation (34).
Previous reports showed that T1D children with autoantibodies have an increase in the Gram-negative Bacteroidetes members, reduction in mucin-degradation and butyrate-producing species (10, 35, 36). Butyrate has an anti-inflammatory effect, induces T regulatory cells in the gut mucosa, and enhances the barrier via tight-junctions expression (37, 38). In addition, researchers demonstrated that T1D children exhibit diminished abundance of lactate-producing bacteria, including B. longum, subspecies infantis. Bifidobacterium species can exert several functions, such as carbohydrate fermentation, acetate and lactate generation, polyphenols and linoleic acids release, and antioxidant activities (39). They also play an important role in the gastrointestinal lymphoid tissue maturation in early life and offer protection against pathogens by bacteriocin release, decrease in luminal pH, and inhibition of epithelial adhesion (40). In agreement with these studies, we detected the prevalence of Gram-negative bacteria in stool samples from our T1D patients and decreased butyrate-producing bacteria, such as Bifidobacterium and Roseburia species, and decreased Clostridium members that could induce anti-inflammatory IL-10 cytokine and T regulatory cells (41).
The germ-free NOD mice have a similar metabolite profile to that of pre-diabetic children, with reduced glycemic control and deregulated immunologic and metabolic responses (42). The absence of a gut microbiota in this mice did not affect the diabetes incidence but promoted insulitis and increased levels of pro-inflammatory IFN-γ and IL-12, suggesting the important role of the microbiota in glucose metabolism (42). Our data suggest that T1D patients with intestinal dysbiosis, with decreased abundance of beneficial microbes, such as Bifidobacterium and Lactobacillales members have poor glycemic control, represented by high levels of HbA1c. The standard method to monitor glycemic control is the measurements of the HbA1c, and the good glycemic control is related to decreased microvascular complications in T1D patients (3, 43, 44). The poor glycemic control is defined as HbA1c between 59 and 106 mmol/mol or 7.5–12% (43). About 25% of T1D patients’ population is in persistent poor glycemic control (44), which is in accordance with our present study, that 13 patients (65%) presented poor glycemic control. Our hypothesis relies on that intestinal dysbiosis and bacterial translocation can induce systemic inflammation and suppression of insulin receptors, promoting an increased glucose blood levels and high levels of HbA1c in T1D patients. Gram-negative-derived LPS binds to TLR4 and trigger the inflammatory cascade, resulting in NF-κB activation and secretion of inflammatory mediators, such as TNF, IL-1, and IL-6, which influence glucose metabolism and inhibit phosphorylation of insulin receptors (45–47).
In the present study, T1D patients presented increased inflammatory IL-6 concentrations that inversely correlated with Ruminococcaceae family, an anaerobe microorganism that degrade complex carbohydrates, and are common in subjects with carbohydrate-enriched diets (48). The inflammatory TNF inversely correlated with Clostridiaceae members that include Clostridium species, which can induce T regulatory cells in the gut mucosa. The Clostridium species, especially clusters IV and XIVa, are spore-forming members from the gut microbiota and can induce T regulatory cells differentiation in the gut mucosa (41).
Proposed mechanisms to explain the link between intestinal dysbiosis and T1D include the dysbiosis-associated immunological deregulation, leading to destruction of β-cells by autoreactive T cells (14, 49). The second proposed mechanism correlates T1D with leaky gut, bacterial translocation, endotoxemia, and chronic low-grade inflammation (12, 50). The Gram-negative bacterial species, prevalent in stool samples from our T1D patients, enhance gut permeability and increase bacterial translocation, which can lead to systemic inflammation and metabolic deregulation (14, 50).
Finally, we concluded that there are different gut microbiota profiles between T1D patients and healthy controls. The prevalent Gram-negative species in T1D patients could be involved in the leaky gut, bacterial translocation, and poor glycemic control. However, additional studies, with larger cohorts, are required to determine a “signature” of the intestinal microbiota in T1D patients in the Brazilian population.
Ethics Statement
The present study was performed in accordance with the recommendations of Ethics committee from Barretos Cancer Hospital. All subjects provided written informed consent in accordance with the Declaration of Helsinki. The protocol was approved by the Barretos Cancer Hospital (Process number 903/2014).
Author Contributions
BH: T1D patients’ enrollment, DNA extraction, cytokine determination, data acquisition, and manuscript writing; MG: controls’ enrollment and sample collection; NR and NS: V3/V4 amplification, library construction, and sequencing; JB: support for blood samples collection; EM: support to Illumina platform sequencing; JP: responsible for clinical data from T1D patients. WO and DP: bioinformatics analyses; VM: sample collection, DNA quantification, and cytokine determination; GO: experimental design, data interpretation, manuscript writing, and revision.
Conflict of Interest Statement
The authors declare that the research was conducted in the absence of any commercial or financial relationships that could be construed as a potential conflict of interest.
Funding
The study was supported by Foundation for the Support of Research in the State of São Paulo (FAPESP, grant numbers #2016/50204-0 and #2018/13702-7) and School of Health Sciences from Barretos Dr. Paulo Prata (PAP#2016).
References
1. Hara N, Alkanani AK, Ir D, Robertson CE, Wagner BD, Frank DN, et al. The role of the intestinal microbiota in type 1 diabetes. Clin Immunol (2013) 146:112–9. doi:10.1016/j.clim.2012.12.001
2. Atkinson MA, Chervonsky A. Does the gut microbiota have a role in type 1 diabetes? Early evidence from humans and animal models of the disease. Diabetologia (2012) 55:2868–77. doi:10.1007/s00125-012-2672-4
3. International Diabetes Federation. International Diabetes Federation Atlas. 8th ed. London: ACW.uk.com (2017).
4. Knip M, Honkanen J. Modulation of type 1 diabetes risk by the intestinal microbiome. Curr Diab Rep (2017) 17:105. doi:10.1007/s11892-017-0933-9
5. Roesch LF, Lorca GL, Casella G, Giongo A, Naranjo A, Pionzio AM, et al. Culture-independent identification of gut bacteria correlated with the onset of diabetes in a rat model. ISME J (2009) 3:536–48. doi:10.1038/ismej.2009.5
6. Bosi E, Molteni L, Radaelli MG, Folini L, Fermo I, Bazzigaluppi E, et al. Increased intestinal permeability precedes clinical onset of type 1 diabetes. Diabetologia (2006) 49:2824–7. doi:10.1007/s00125-006-0465-3
7. Vaarala O. Human intestinal microbiota and type 1 diabetes. Curr Diab Rep (2013) 13:601–7. doi:10.1007/s11892-013-0409-5
8. Giongo A, Gano KA, Crabb DB, Mukherjee N, Novelo LL, Casella G, et al. Toward defining the autoimmune microbiome for type 1 diabetes. ISME J (2011) 5:82–91. doi:10.1038/ismej.2010.92
9. Murri M, Leiva I, Gomez-Zumaquero JM, Tinahones FJ, Cardona F, Soriquer F, et al. Gut microbiota in children with type 1 diabetes differs from that in healthy children: a case-control study. BMC Med (2013) 11:46. doi:10.1186/1741-7015-11-46
10. De Goffau MC, Luopajärvi K, Knip M, Ilonen J, Ruohtula T, Härkönen T, et al. Fecal microbiota composition differs between children with β-cell autoimmunity and those without. Diabetes (2013) 62:1238–44. doi:10.2337/db12-0526
11. Davis-Richardson AG, Ardissone AN, Dias R, Simell V, Leonard MT, Kemppainen KM, et al. Bacteroides dorei dominates gut microbiome prior to autoimmunity in Finnish children at high risk for type 1 diabetes. Front Microbiol (2014) 5:678. doi:10.3389/fmicb.2014.00678
12. Li X, Atkinson MA. The role for gut permeability in the pathogenesis of type 1 diabetes – a solid or leaky concept? Pediatr Diabetes (2015) 16:485–92. doi:10.1111/pedi.12305
13. Meiía-León ME, Petrosino JF, Aiami NJ, Domínguez-Bello MG, De La Barca AM. Fecal microbiota imbalance in Mexican children with type 1 diabetes. Sci Rep (2014) 4:3814. doi:10.1038/srep03814
14. Sohail MU, Althani A, Anwar H, Rizzi R, Marei HE. Role of the gastrointestinal tract microbiome in the pathophysiology of diabetes mellitus. J Diabetes Res (2017) 2017:9631435. doi:10.1155/2017/9631435
15. Sargent J. Diabetes. Altered gut microbial networks linked to islet cell autoimmunity. Nat Rev Endocrinol (2014) 10:313. doi:10.1038/nrendo.2014.43
16. Sommer F, Anderson JM, Bharti R, Raes J, Rosenstiel P. The resilience of the intestinal microbiota influences health and disease. Nat Rev Microbiol (2017) 15:630–8. doi:10.1038/nrmicro.2017.58
17. Klindworth A, Pruesse E, Schweer T, Peplies J, Quast C, Horn M, et al. Evaluation of general 16S ribosomal RNA gene PCR primers for classical and next-generation sequencing-based diversity studies. Nucleic Acids Res (2013) 41:e1. doi:10.1093/nar/gks808
18. Leite AZ, Rodrigues NC, Gonzaga MI, Paiolo JCC, de Souza CA, Stefanutto NAV, et al. Detection of increased plasma interleukin-6 levels and prevalence of Prevotella copri and Bacteroides vulgatus in the feces of type 2 diabetes patients. Front Immunol (2017) 8:1107. doi:10.3389/fimmu.2017.01107
19. McDermott AJ, Huffnagle GB. The microbiome and regulation of mucosal immunity. Immunology (2014) 142:24–31. doi:10.1111/imm.12231
20. Sommer F, Bäckhed F. The gut microbiota – masters of host development and physiology. Nat Rev Microbiol (2013) 11:227–38. doi:10.1038/nrmicro2974
21. Biedermann L, Rogler G. The intestinal microbiota: its role in health and disease. Eur J Pediatr (2015) 174:151–67. doi:10.1007/s00431-014-2476-2
22. Wu H, Tremaroli V, Bäckhed F. Linking microbiota to human diseases: a systems biology perspective. Trends Endocrinol Metab (2015) 26:758–70. doi:10.1016/j.tem.2015.09.011
23. Knip M, Siljander H. The role of the intestinal microbiota in type 1 diabetes mellitus. Nat Rev Endocrinol (2016) 12:154–67. doi:10.1038/nrendo.2015.218
24. Lerner A, Aminov R, Matthias T. Dysbiosis may trigger autoimmune diseases via inappropriate post-translational modification of host proteins. Front Microbiol (2016) 7:84. doi:10.3389/fmicb.2016.00084
25. Doré J, Blottière H. The influence of diet on the gut microbiota and its consequences for health. Curr Opin Biotechnol (2015) 32:195–9. doi:10.1016/j.copbio.2015.01.002
26. Moschen AR, Wieser V, Tilg H. Dietary factors: major regulators of the gut’s microbiota. Gut Liver (2012) 6:411–6. doi:10.5009/gnl.2012.6.4.411
27. Wu GD, Chen J, Hoffman C, Bittinger K, Chen YY, Keilbaugh SA, et al. Linking long-term dietary patterns with gut microbial enterotypes. Science (2011) 334:105–8. doi:10.1126/science.1208344
28. Yamaguchi Y, Adachi K, Sugiyama T, Shimozato A, Ebi M, Ogasawara N, et al. Association of intestinal microbiota with metabolic markers and dietary habits in patients with type 2 diabetes. Digestion (2016) 94:66–72. doi:10.1159/000447690
29. Costa FR, Françozo MC, de Oliveira GG, Ignacio A, Castoldi A, Zamboni DS, et al. Gut microbiota translocation to the pancreatic lymph nodes triggers NOD2 activation and contributes to T1D onset. J Exp Med (2016) 213:1223–39. doi:10.1084/jem.20150744
30. Eckburg PB, Bik EM, Bernstein CN, Purdom E, Dethlefsen L, Sargent M, et al. Diversity of the human intestinal microbial flora. Science (2005) 308:1635–8. doi:10.1126/science.1110591
31. Kelly CJ, Zheng L, Campbell EL, Saeedi B, Scholz CC, Bavless AJ, et al. Crosstalk between microbiota-derived short-chain fatty acids and intestinal epithelial HIF augments tissue barrier function. Cell Host Microbe (2015) 17:662–71. doi:10.1016/j.chom.2015.03.005
32. Canabi RB, Costanzo MD, Leone L, Pedata M, Meli R, Calignano A. Potential beneficial effects of butyrate in intestinal and extraintestinal diseases. World J Gastroenterol (2011) 17:1519–28. doi:10.3748/wjg.v17.i12
33. Wexler HM. Bacteroides: the good, the bad, and the nitty-gritty. Clin Microbiol Rev (2007) 20:593–621. doi:10.1128/CMR.00008-07
34. Larsen JM. The immune response to Prevotella bacteria in chronic inflammatory disease. Immunology (2017) 151:363–74. doi:10.1111/imm.12760
35. De Goffau MC, Fuentes S, Van Den Bogert B, Honkanen H, De Vos WM, Welling GW, et al. Aberrant gut microbiota composition at the onset of type 1 diabetes in young children. Diabetologia (2014) 57:1569–77. doi:10.1007/s00125-014-3274-0
36. Brown CT, Davis-Richardson AG, Giongo A, Gano KA, Crabb DB, Mukherjee N, et al. Gut microbiome metagenomics analysis suggests a functional model for the development of autoimmunity for type 1 diabetes. PLoS One (2011) 6:e25792. doi:10.1371/journal.pone.0025792
37. Arpaia N, Campbell C, Fan X, Dikiv S, Van der Veeken J, De Roos P, et al. Metabolites produced by commensal bacteria promote peripheral regulatory T-cell generation. Nature (2013) 504:451–5. doi:10.1038/nature12726
38. Furusawa Y, Obata Y, Fukuda S, Endo TA, Nakato G, Takahashi D, et al. Commensal microbe-derived butyrate induces the differentiation of colonic regulatory T cells. Nature (2013) 504:446–50. doi:10.1038/nature12721
39. Endesfelder D, Zu Castell W, Ardissone A, Davis-Richardson AG, Achenbach P, Hagen M, et al. Compromised gut microbiota networks in children with anti-islet cell autoimmunity. Diabetes (2014) 63:2006–14. doi:10.2337/db13-1676
40. Rivière A, Selak M, Lantin D, Leroy F, De Vuyst L. Bifidobacteria and butyrate-producing colon bacteria: importance and strategies for their stimulation in the human gut. Front Microbiol (2016) 7:979. doi:10.3389/fmicb.2016.00979
41. Atarashi K, Tanoue T, Shima T, Imaoka A, Kuwahara T, Momose Y, et al. Induction of colonic regulatory T cells by indigenous Clostridium species. Science (2011) 331:337–41. doi:10.1126/science.1198469
42. Greiner TU, Hyötyläinen T, Knip M, Bäckhed F, Orešič M. The gut microbiota modulates glycaemic control and serum metabolite profiles in non-obese diabetic mice. PLoS One (2014) 9:e110359. doi:10.1371/journal.pone.0110359
43. Leelarathna L, Guzder R, Muralidhara K, Evans ML. Diabetes: glycaemic control in type 1. BMJ Clin Evid (2011) 5:607.
44. Devries JH, Snoek FJ, Heine RJ. Persistent poor glycaemic control in adult type 1 diabetes. A closer look at the problem. Diabet Med (2004) 21:1263–8. doi:10.1111/j.1464-5491.2004.01386.x
45. Cani PD, Bibiloni R, Knauf C, Waget A, Neyrinck AM, Delzenne NM, et al. Changes in gut microbiota control metabolic endotoxemia-induced inflammation in high-fat diet-induced obesity and diabetes in mice. Diabetes (2008) 57:1470–81. doi:10.2337/db07-1403
46. Caricilli AM, Saad MJ. The role of gut microbiota on insulin resistance. Nutrients (2013) 5:829–51. doi:10.3390/nu5030829
47. Cani PD, Neyrinck AM, Fava F, Knauf C, Burcelin RG, Tuohy KM, et al. Selective increases of bifidobacteria in gut microflora improve high fat-diet-induced diabetes in mice through a mechanism associated with endotoxemia. Diabetologia (2007) 50:2374–83. doi:10.1007/s00125-007-0791-0
48. La Reau AJ, Meier-Kolthoff JP, Suen G. Sequence-based analysis of the genus Ruminococcus resolves its phylogeny and reveals strong host association. Microb Genom (2016) 2:e000099. doi:10.1099/mgen.0.000099
49. Levy M, Kolodziejczyk AA, Thaiss CA, Elinav E. Dysbiosis and the immune system. Nat Rev Immunol (2017) 17:219–32. doi:10.1038/nri.2017.7
Keywords: type 1 diabetes, dietary habits, intestinal dysbiosis, inflammatory cytokines, interleukin-6, glycemic control
Citation: Higuchi BS, Rodrigues N, Gonzaga MI, Paiolo JCC, Stefanutto N, Omori WP, Pinheiro DG, Brisotti JL, Matheucci E Jr., Mariano VS and de Oliveira GLV (2018) Intestinal Dysbiosis in Autoimmune Diabetes Is Correlated With Poor Glycemic Control and Increased Interleukin-6: A Pilot Study. Front. Immunol. 9:1689. doi: 10.3389/fimmu.2018.01689
Received: 06 April 2018; Accepted: 10 July 2018;
Published: 25 July 2018
Edited by:
Juarez Antonio Simões Quaresma, Instituto Evandro Chagas, BrazilReviewed by:
Maryam Dadar, Razi Vaccine and Serum Research Institute, IranLidia Santarpia, Università degli Studi di Napoli Federico II, Italy
Alexander Nikolaevich Orekhov, Institute for Atherosclerosis Research, Russia
Copyright: © 2018 Higuchi, Rodrigues, Gonzaga, Paiolo, Stefanutto, Omori, Pinheiro, Brisotti, Matheucci, Mariano and de Oliveira. This is an open-access article distributed under the terms of the Creative Commons Attribution License (CC BY). The use, distribution or reproduction in other forums is permitted, provided the original author(s) and the copyright owner(s) are credited and that the original publication in this journal is cited, in accordance with accepted academic practice. No use, distribution or reproduction is permitted which does not comply with these terms.
*Correspondence: Gislane Lelis Vilela de Oliveira, glelisvilela@gmail.com